
Harnessing Artificial Intelligence for High-Impact Science
Particle colliders reveal details of fundamental properties of matter that not only satisfy our curiosity about the universe, but also allow us to build better technologies. Unfortunately, these discoveries can take decades because collision experiments generate enormous datasets, and scientists must parse through the wealth of different measurements that are taken by sensitive detectors every time particles are smashed together.
Our scientists developed a machine learning tool called OmniFold to make the data sorting and analysis process easier, and used it to study the inner structure of protons using data from the HERA (Hadron–Electron Ring Accelerator) collider at the Deutsches Elektronen-Synchrotron (DESY) in Germany.
OmniFold can run analyses that used to take years in mere minutes. It runs on supercomputers like Perlmutter, the flagship system at Berkeley Lab’s National Energy Research Scientific Computing Center (NERSC). Perlmutter and other NERSC systems provide world-leading computing resources to support DOE research. The team hopes to apply OmniFold to future particle datasets, including those generated at DOE’s Electron-Ion Collider (EIC), which will be built at Brookhaven National Laboratory in partnership with Thomas Jefferson National Accelerator Facility. Berkeley Lab will contribute to the accelerator and detector of the EIC.
In another AI-powered contribution to particle studies, a Berkeley Lab team developed an award-winning machine learning tool that can find hidden patterns in particle accelerator datasets. Their code was developed for (and won) an international particle physics challenge, and has relevant applications in other areas as well, such as the search for transient or anomalous signals in astrophysics research. It now helps physicists find evidence of unknown particles that might otherwise be overlooked when performing traditional analyses.
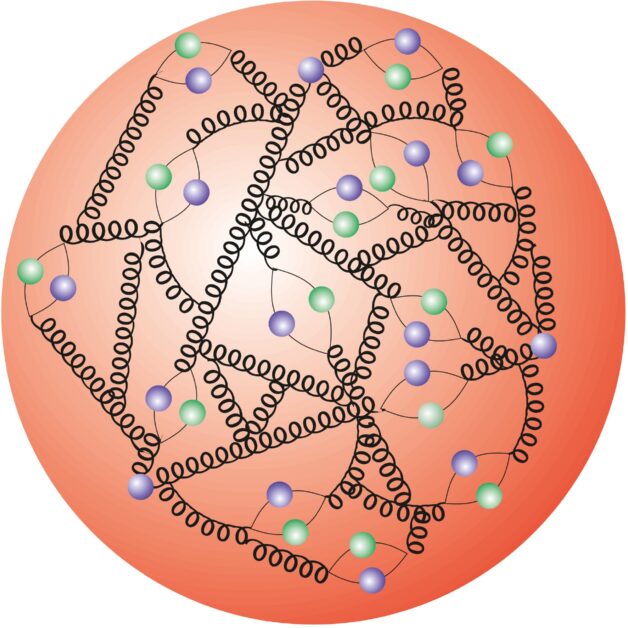
An illustration of a proton with its constituent parts: quarks (shown as green and purple balls in the illustration above) and gluons (illustrated as black coils). Scientists can investigate these parts by studying collisions between electrons and protons.
gpCAM is a powerful tool developed by the Center for Advanced Mathematics for Energy Research Applications (CAMERA) at Berkeley Lab that automates the process of collecting and analyzing data in scientific experiments. By using advanced mathematical methods, it helps researchers quickly and accurately predict outcomes and measure uncertainty in large datasets, making experiments faster and more efficient. This automation enhances the overall effectiveness and precision of scientific investigations across various fields. Today, gpCAM is used across the U.S. and Europe for autonomous data acquisition in simulations and experiments.
Recently, a Berkeley Lab team used gpCAM to study the quantum properties of 2D materials, which reduced microscopy imaging time from three weeks down to 8 hours. 2D materials have a variety of applications as components of transistors, semiconductors, batteries, lasers, and camera sensors, as well as uses in drug delivery and water purification. Researchers at Brookhaven National Laboratory and the Institut Laue-Langevin have also leveraged gpCAM to optimize data acquisition in X-ray microscopy and neutron scattering experiments, respectively, significantly speeding up the collection of high-quality data and improving experimental workflows. gpCAM is open source and available to the scientific community. In 2024, it received an R&D 100 Award for its significant contribution to scientific research and innovation.

Distribution channels: Science
Legal Disclaimer:
EIN Presswire provides this news content "as is" without warranty of any kind. We do not accept any responsibility or liability for the accuracy, content, images, videos, licenses, completeness, legality, or reliability of the information contained in this article. If you have any complaints or copyright issues related to this article, kindly contact the author above.
Submit your press release